The Role of Artificial Intelligence in Personalized Health Monitoring Systems
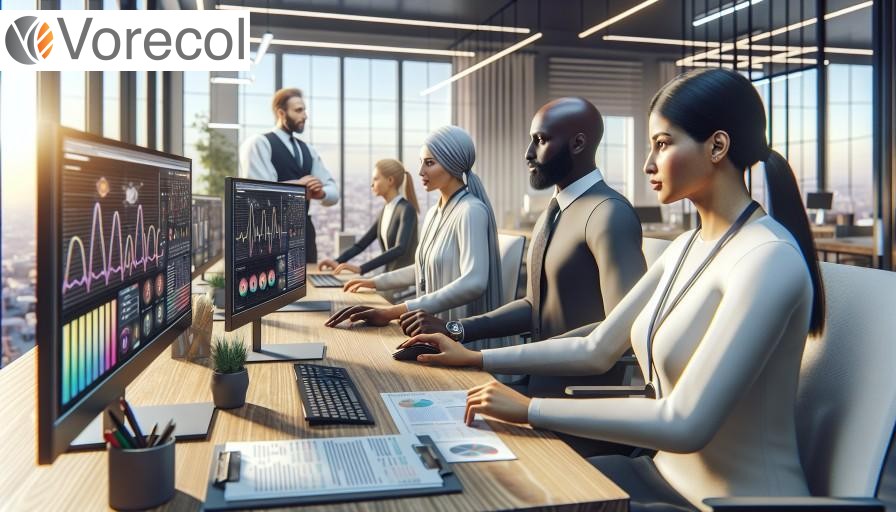
- 1. Introduction to Personalized Health Monitoring Systems
- 2. Understanding the Basics of Artificial Intelligence
- 3. How AI Enhances Data Collection and Analysis
- 4. Machine Learning Algorithms in Health Monitoring
- 5. Real-time Health Tracking and Predictive Analytics
- 6. Ethical Considerations in AI-Driven Health Systems
- 7. Future Trends in AI and Personalized Health Monitoring
- Final Conclusions
1. Introduction to Personalized Health Monitoring Systems
In a world increasingly driven by data, personalized health monitoring systems are revolutionizing how we maintain and manage our well-being. Take the story of Livongo, a digital health company that empowers individuals with chronic conditions. With over 1.5 million members, their platform uses real-time data to deliver personalized insights and coaching. Patients with diabetes receive constant monitoring, which not only helps in lowering their A1C levels by an average of 1.5 points but also promotes proactive behavior. This innovative approach is enabling people to take control of their health journey, highlighting the importance of utilizing technology to tailor healthcare to individual needs.
As organizations pivot to embrace personalized health monitoring, there are key practices to consider. A recommendation from the case of Oura, a health tech company known for its smart ring, is to integrate user feedback directly into product development. Oura’s commitment to listening to its community has resulted in an 80% increase in user engagement over the past year. For those developing similar systems, it’s essential to ensure privacy and data security, as highlighted by the backlash faced by companies like Theranos. Prioritizing user trust through transparent data practices can lead to higher adoption rates and ultimately, better health outcomes.
2. Understanding the Basics of Artificial Intelligence
Artificial Intelligence (AI) is a revolutionary force that is reshaping industries and transforming our daily lives. A striking example is IBM's Watson, which gained fame for defeating human champions on the game show "Jeopardy!" But its real impact extends beyond entertainment; in healthcare, Watson now analyzes vast amounts of medical data and helps oncologists make more accurate treatment decisions. According to a report by McKinsey, the healthcare sector could save up to $150 billion annually by implementing AI solutions. For organizations looking to integrate AI into their operations, starting with pilot projects that focus on data-driven decision-making can yield quick wins. Companies should also invest in skilled talent capable of interpreting AI outputs to ensure they are used effectively.
Meanwhile, in the world of retail, Amazon has harnessed AI to personalize customer experiences, employing algorithms that predict what customers may want based on their browsing history. This strategy contributed to Amazon's staggering 38% revenue growth in 2020 alone. As businesses aim to leverage AI, a practical recommendation is to embrace a culture of experimentation; this encourages teams to test different AI applications while closely monitoring performance metrics. Additionally, organizations must prioritize data quality by establishing robust data management practices. By understanding these foundational elements of AI, companies can unlock new opportunities for innovation and efficiency in a constantly evolving marketplace.
3. How AI Enhances Data Collection and Analysis
In a bustling city, a small retail chain named Zara shifted gears by implementing AI-powered analytics to understand customer preferences. By integrating machine learning algorithms into their point of sale systems, they began collecting data that revealed purchasing patterns and seasonal trends. Within just six months, Zara saw a remarkable 30% increase in sales, attributed to their ability to stock the right products at the right time. This success story highlights how AI can revolutionize data collection when businesses harness these technologies to make informed decisions. For organizations looking to emulate Zara's success, investing in AI-driven tools that streamline data analysis can provide insights that traditional methods might overlook.
Meanwhile, the healthcare sector is experiencing a similar transformation. By using AI to analyze patient data, organizations like IBM Watson Health have established predictive models that can estimate patient outcomes with startling accuracy. For instance, Watson's AI was able to analyze the genetic makeup of cancer patients and predict the best course of treatment, significantly improving patient care. This case underscores the necessity of not just collecting data but also deploying AI to derive actionable insights. Organizations striving for similar outcomes should prioritize the integration of AI into their data collection strategies and focus on training their staff to utilize these tools effectively, ensuring that every data point is a building block towards better decision-making.
4. Machine Learning Algorithms in Health Monitoring
In the realm of health monitoring, machine learning algorithms are transforming how we prevent and manage diseases. Take for instance the case of Tempus, a technology company that harnesses machine learning to analyze clinical and molecular data. By fine-tuning algorithms that predict treatment outcomes for cancer patients, Tempus has been able to provide physicians with actionable insights, leading to more personalized therapies. According to their metrics, these algorithms have helped increase the accuracy of treatment decisions by over 30%. For healthcare organizations looking to implement machine learning, starting with clean, well-organized data and collaborating with data scientists can significantly improve the effectiveness of predictive models.
Another compelling example comes from the wearable technology company Fitbit, which uses machine learning to analyze users’ health data to forecast health risks and suggest lifestyle changes. Their algorithms can detect anomalies in heart activity, alerting users to potential health issues before they escalate into serious conditions. Fitbit's approach demonstrates the importance of user engagement: by encouraging users to regularly track their fitness and health metrics, they not only gather valuable data but also empower individuals to take charge of their health. For those in similar situations, leveraging user data through intuitive platforms and regular feedback mechanisms can create a proactive health monitoring system that translates data into meaningful health outcomes.
5. Real-time Health Tracking and Predictive Analytics
In 2018, the healthcare startup Livongo emerged as a powerful force in real-time health tracking, focusing on chronic condition management. Through its innovative platform, Livongo provided users with connected devices and real-time data analytics, enabling individuals with diabetes to monitor their blood glucose levels effortlessly. By integrating predictive analytics, the platform offered insights into potential health risks, allowing for timely interventions. Within just two years, Livongo reported a 30% reduction in hospital admissions for its users, highlighting the profound impact of real-time tracking on patient outcomes. For those looking to implement similar strategies, investing in user-friendly technology is essential, as it ensures greater engagement and compliance. Pairing this with robust analytical capabilities will allow organizations to anticipate patient needs and create tailored interventions efficiently.
On the global stage, organizations like Apple have incorporated health tracking through their devices, offering users features to monitor heart rate, activity levels, and even ECG readings. By harnessing predictive analytics, Apple's Health app can alert users about irregularities that might require medical attention, thereby empowering users to take charge of their health proactively. A growing number of studies indicate that early detection of health issues can lead to more favorable outcomes, with preventative measures reducing the likelihood of chronic diseases by nearly 70%. For healthcare providers or organizations, fostering partnerships with tech companies can be instrumental in accessing state-of-the-art tools for patient engagement. Additionally, training staff on utilizing these systems will create a culture of data-driven decision-making, ensuring that predictive analytics are effectively integrated into patient care.
6. Ethical Considerations in AI-Driven Health Systems
In 2019, the University of California, San Francisco (UCSF) implemented an AI-driven tool aimed at predicting patient deterioration in intensive care units. While the technology was revolutionary, it also sparked ethical debates about data privacy and bias, particularly as it utilized historical health data that may not have represented all demographic groups equally. For instance, the algorithm performed poorly for minority communities, raising concerns about unequal access to interventions. To counteract such challenges, organizations must adopt a framework that emphasizes fairness, accountability, and transparency in AI systems. Establishing diverse teams during the development phase not only mitigates bias but also enriches the understanding of varied patient needs, ensuring a more inclusive healthcare solution.
Similarly, a notable case occurred in the UK when the National Health Service (NHS) partnered with DeepMind to develop an AI system for detecting eye disease. Initially heralded as a monumental leap forward in healthcare, it faced scrutiny regarding the ethical management of patient data, leading to a temporary halt in the project. This situation illuminated the importance of informed consent and open communication with patients about how their health data would be used. As a practical recommendation, healthcare organizations should proactively engage patients and stakeholders in discussions about AI technologies, prioritizing transparency and user-centric data practices. By fostering this trust, healthcare providers can create a more supportive environment while harnessing the transformative potential of AI in health systems.
7. Future Trends in AI and Personalized Health Monitoring
In a world increasingly driven by data, the intersection of artificial intelligence and personalized health monitoring is set to revolutionize the way we perceive healthcare. Consider the case of IBM Watson Health, which harnesses AI algorithms to analyze medical data, enabling tailored healthcare solutions for patients. This innovative technology has shown a 30% improvement in treatment outcomes for patients with certain types of cancer, as it provides physicians with actionable insights based on vast datasets. For individuals navigating the complex landscape of health challenges, leveraging AI-powered platforms like MySugr can offer real-time glucose monitoring and personalized feedback, effectively empowering patients to manage diabetes proactively. As these technologies evolve, they create a narrative where health management becomes a collaborative journey between patient and AI.
However, the embrace of AI in health monitoring is not without its challenges. Companies like Apple have begun to incorporate advanced health tracking features in their devices, but privacy concerns remain a significant hurdle, with 61% of users expressing anxiety about how their data is being used. To navigate this terrain, individuals should seek out platforms prioritizing transparency and data security. Opting for services that provide clear information on data usage while allowing users control over their health information can foster trust. Additionally, healthcare professionals must be well-versed in these tools to effectively incorporate them into patient care. As we look to the future, the promise of AI and personalized health monitoring lies not just in its capabilities, but in the responsible and ethical approach taken by both companies and individuals alike.
Final Conclusions
In conclusion, the integration of artificial intelligence in personalized health monitoring systems represents a transformative shift in healthcare delivery. By harnessing the power of AI, these systems enable continuous and real-time assessment of individual health metrics, allowing for tailored interventions that can prevent diseases and enhance overall well-being. The ability to analyze large volumes of data from various sources not only supports personalized treatment plans but also empowers patients to take an active role in their health journey. As AI technologies continue to evolve, their application in health monitoring will likely become increasingly sophisticated, offering unprecedented opportunities for early detection and personalized care.
Moreover, the potential challenges associated with the implementation of AI in personalized health monitoring must be acknowledged and addressed. Ethical considerations, data privacy concerns, and the need for robust regulatory frameworks are critical to ensuring that these technologies benefit all individuals without exacerbating existing healthcare disparities. As stakeholders from both the technology and healthcare sectors collaborate to navigate these complexities, the promise of AI-driven health monitoring systems can be fully realized, paving the way for a more proactive, patient-centered approach to healthcare that ultimately improves health outcomes and quality of life for all.
Publication Date: August 29, 2024
Author: Psico-smart Editorial Team.
Note: This article was generated with the assistance of artificial intelligence, under the supervision and editing of our editorial team.
💡 Would you like to implement this in your company?
With our system you can apply these best practices automatically and professionally.
Work Environment - Climate Assessment
- ✓ Measure and improve your work climate
- ✓ Detailed surveys + comparative analysis
✓ No credit card ✓ 5-minute setup ✓ Support in English
💬 Leave your comment
Your opinion is important to us